HOME | DD
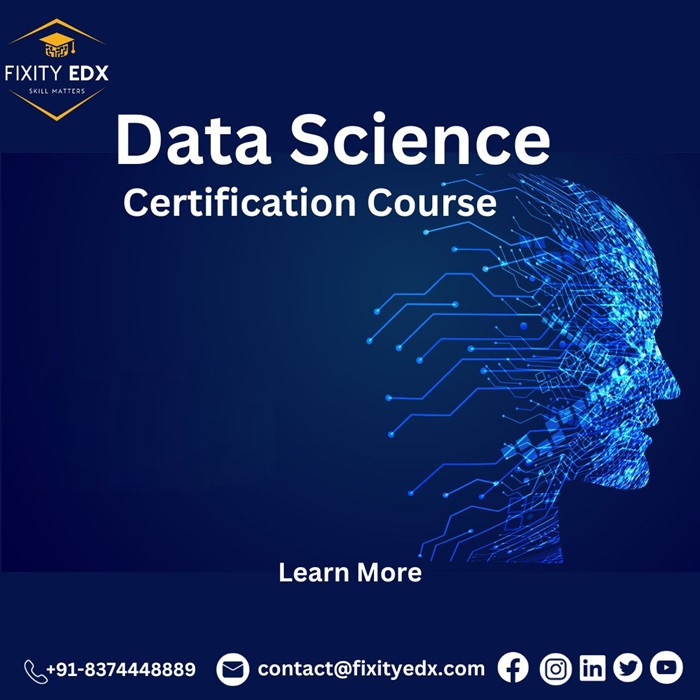
Published: 2023-10-27 07:33:34 +0000 UTC; Views: 132; Favourites: 0; Downloads: 0
Redirect to original
Description
Data Science Course Projects: From Theory to Real-World Applications
In the realm of Data science education, theory is undoubtedly crucial. Understanding the underlying principles, algorithms, and methodologies is fundamental to mastering the discipline. However, it's the practical application of this knowledge that truly sets data scientists apart. This is where data science course projects come into play, bridging the gap between theory and real-world applications.
The Importance of Data Science Course ProjectsData science courses often include project work as a significant component, and for good reason. These projects serve several essential purposes:
1. Application of Knowledge:Data science is not just about knowing the theory; it's about using it to solve real-world problems. Course projects provide students with the opportunity to apply the concepts they've learned in a hands-on environment.
2. Skill Development:Projects encourage the development of practical skills, such as data collection, cleaning, analysis, and visualization. These skills are vital in a data scientist's day-to-day work.
3. Portfolio Building:A well-executed course project can become a valuable addition to your professional portfolio. It showcases your abilities to potential employers or collaborators.
4. Problem-Solving:In data science, every project comes with its unique challenges. Students learn how to approach complex problems and find innovative solutions, preparing them for the unpredictability of the real data science world.
Types of Data Science Course ProjectsData science course projects can vary in complexity and scope. Here are some common types:
1. Exploratory Data Analysis (EDA):EDA projects involve thorough data exploration, visualization, and the identification of patterns and trends within the dataset. Students learn to ask the right questions and gain insights from the data.
2. Predictive Modeling:Predictive modeling projects focus on building machine learning models that can make predictions based on historical data. Students work on selecting appropriate algorithms, training models, and evaluating their performance.
3. Natural Language Processing (NLP):NLP projects involve the analysis of text data. Students learn to process and extract meaningful information from textual content, which has applications in sentiment analysis, chatbots, and more.
4. Image Recognition:These projects revolve around image data and often include tasks like object detection, classification, or image generation using techniques like convolutional neural networks (CNNs).
5. Time Series Analysis:Time series projects involve analyzing data points collected over time, which is crucial in fields such as finance, epidemiology, and climate science.
Real-World ImpactWhat truly sets data science course projects apart is their potential for real-world impact. Students often work on projects with practical applications, which may include:
Predictive Maintenance: Developing models to predict when machines or equipment may fail, helping businesses avoid costly downtime.
Healthcare: Analyzing patient data to predict disease outcomes or optimize treatment plans.
E-commerce: Recommender systems that enhance user experience and increase sales.
Financial Analysis: Predicting market trends, managing risk, and optimizing investment portfolios.
It's important to acknowledge that course projects aren't without challenges. Students may encounter issues with data quality, limited resources, or complex project requirements. However, these challenges mirror the realities of the data science profession. Overcoming them not only demonstrates technical competence but also problem-solving abilities.
In conclusion, Data science course projects play a pivotal role in a student's journey from theory to real-world application. They provide opportunities to apply knowledge, develop practical skills, and make a tangible impact. As you embark on your data science education, embrace the challenges of course projects as they are your stepping stones to becoming a proficient and effective data scientist. Remember, it's not just about understanding the theory; it's about putting it into practice to create meaningful change in the world of data science.